AI-Driven Forecasting for Optimal Staffing in Retail Distribution Centers
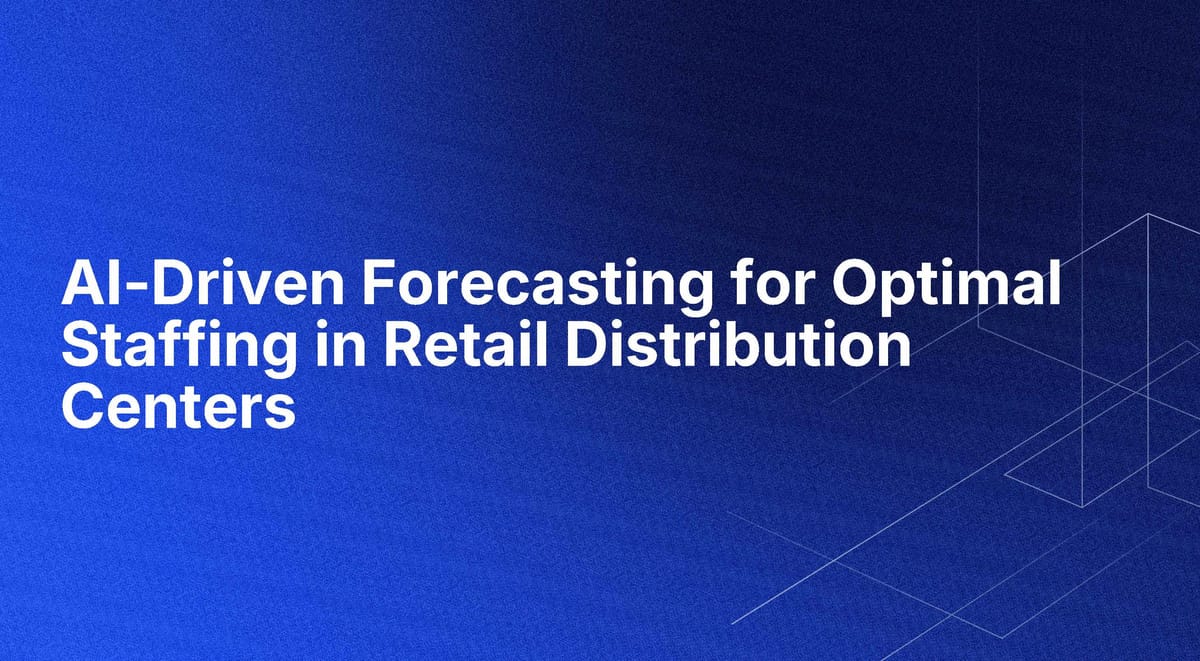
Leveraging AI to Predict Inbound Shipments and Optimize Workforce Management
Overview
The retail and logistics industry is constantly challenged to manage resources efficiently to meet dynamic and often unpredictable demand. Distribution centers (DCs) and warehouses play a crucial role in this ecosystem, acting as hubs where goods are received, processed, and dispatched to various retail locations or directly to customers. Effective management of these facilities is essential for cost containment and customer satisfaction. However, one of the primary obstacles in achieving operational efficiency is the variability in demand, which can affect staffing levels and, consequently, operational costs.
Problem Statement
For companies operating distribution centers or warehouses, utilizing resources efficiently is vital for maintaining smooth supply chain operations. Variability in demand, especially when it can vary by up to 50% daily, makes staffing a significant challenge. Companies are required to notify unionized workforce of their schedules weeks in advance, meaning inaccurate demand forecasting results in either overstaffing or understaffing. Overstaffing leads to underutilized resources and higher operational costs, while understaffing can disrupt the supply chain, causing delays and dissatisfaction among customers.
Solution Overview
AI-driven forecasting provides a sophisticated solution to the problem of staff allocation in distribution centers. Unlike traditional methods, which often rely on linear models and static data, AI leverages large datasets, including historical shipment volumes, seasonal trends, and external factors such as holidays and weather patterns. By processing this complex data, AI can provide highly accurate predictions of inbound shipment volumes over the next month. These precise forecasts allow supply chain managers to make informed staffing decisions, reducing variability and aligning workforce needs with actual demand.