Detect and Classify Product Defects Using AI
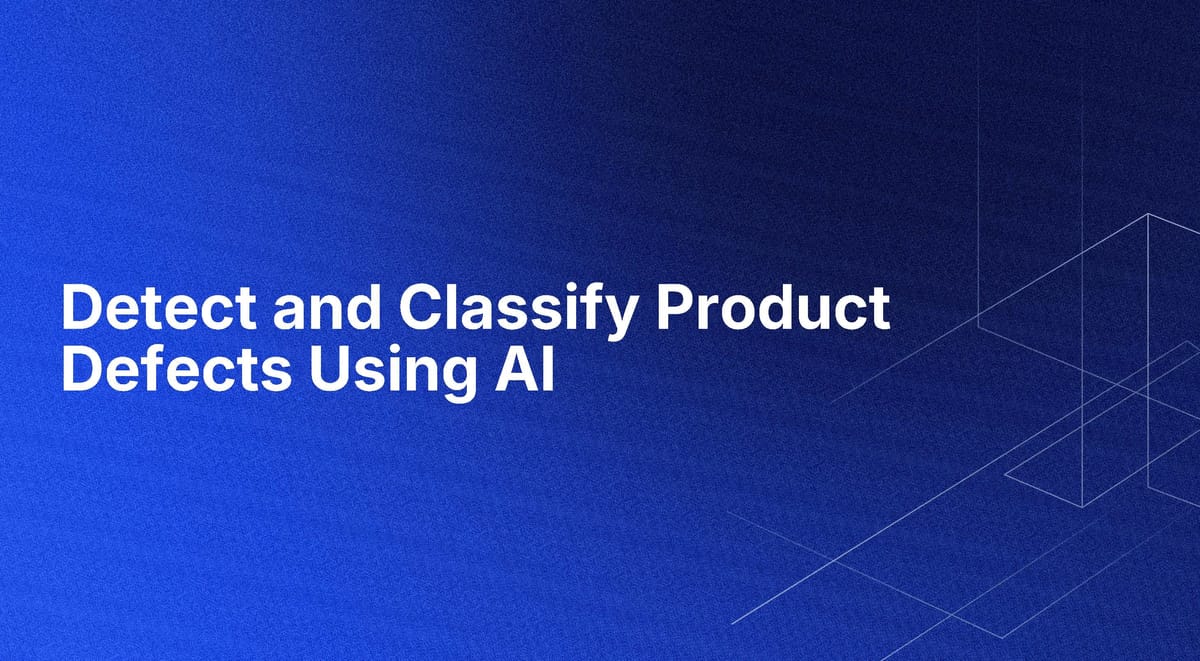
Automate quality assurance and minimize manufacturing costs with AI-powered defect detection
Overview
Manufacturing industries across the globe face numerous challenges, among which maintaining high-quality standards is paramount. From consumer to industrial goods, ensuring that products meet customer expectations requires rigorous quality assurance processes. Defects can range from surface scratches to structural inconsistencies, each impacting the final quality of the product. This is particularly crucial in industries dealing with materials like steel sheets, where surface quality is a critical parameter for the final application.
Problem Statement
Defective materials significantly hinder the manufacturing process, leading to waste, increased production costs, and operational downtimes. The traditional method of defect detection relies heavily on manual inspections, which are time-consuming, labor-intensive, and often prone to human errors. This approach not only inflates costs due to labor and inspection but also contributes to production delays and lost profits from undetected or late-identified defects. The need for a more efficient, accurate, and automated solution is evident.
Solution Overview
Artificial Intelligence (AI), specifically computer vision, offers a transformative approach to identifying and classifying defects in manufacturing processes. Computer vision technology can analyze images of steel sheets to recognize patterns indicative of various defects. By training machine learning models on a dataset containing images of both defective and non-defective steel sheets, the AI can learn to classify defects with high accuracy. This automated inspection minimizes human intervention, reducing labor costs and significantly speeding up the quality assurance process. Implementing this AI solution involves capturing high-quality images of steel sheets and feeding them into a trained computer vision model. The model can then analyze each image in real-time, flagging any defects and classifying them according to their type and severity. Maintenance engineers can monitor the AI system's outputs, ensuring quality levels are met without the need for exhaustive manual inspections. The integration of such AI-driven systems can lead to enhanced efficiency, reduced production downtime, and improved overall product quality. This approach is scalable and can be tailored to other manufacturing processes, making it a versatile tool for various industries aiming to enhance their quality assurance protocols while managing operational costs effectively.