Forecast Daily Demand by Store
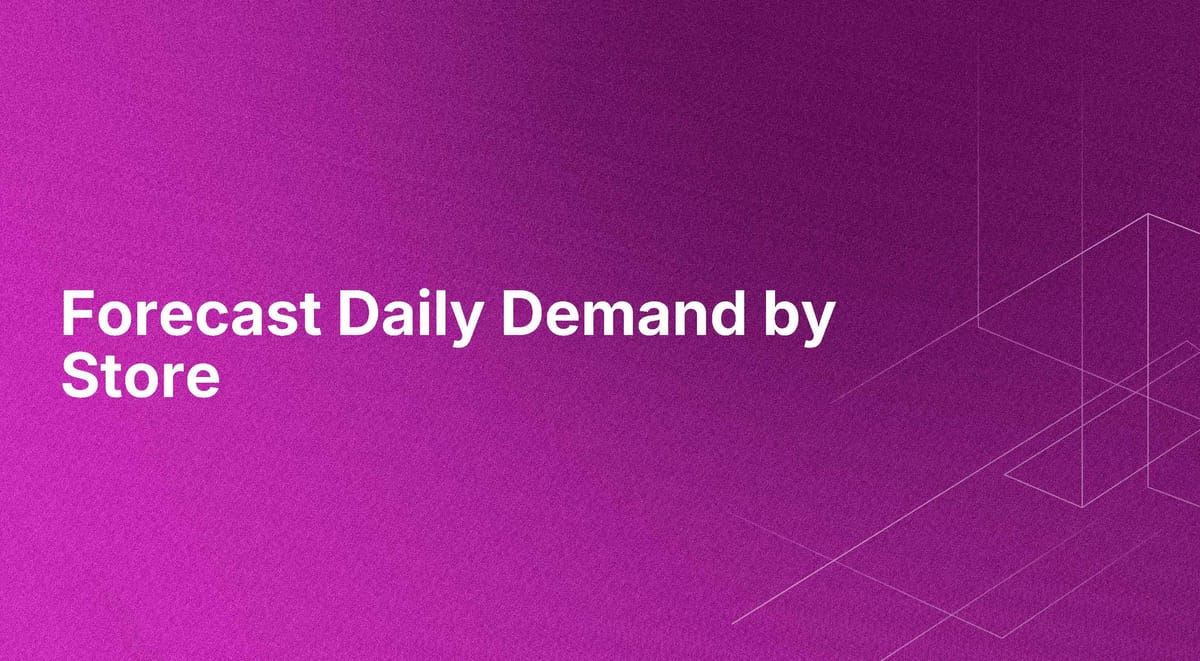
Leverage AI/ML to Optimize Retail Inventory Management
Overview
The retail industry is a dynamic environment characterized by rapid changes in consumer behavior, seasonality, and market trends. With fierce competition and a focus on optimizing consumer satisfaction, retailers strive to balance inventory levels to meet demand without incurring losses through overstock or understock situations. Advanced analytics and AI technologies are becoming pivotal in refining inventory management practices to ensure profitability and customer satisfaction in the retail sector.
Problem Statement
Retailers face the persistent challenge of managing their inventory efficiently. Overstock situations can lead to excessive markdowns and wasted products, while understock scenarios result in missed sales opportunities and customer dissatisfaction. Traditional forecasting methods often fall short due to their inability to account for nuanced data patterns brought on by holidays, special events, and promotional activities. This limitation hinders the ability to create accurate, granular demand forecasts at the product and store levels.
Solution Overview
The solution leverages advanced machine learning and deep learning algorithms to forecast demand with greater accuracy, thus mitigating the challenges of both overstock and understock. These models can scale to handle vast amounts of data, making it possible to generate detailed forecasts for up to a million different product series within a single project. This is particularly advantageous in retail settings where diverse product lines exhibit unique sales patterns shaped by various factors, including seasonality and consumer behavior trends. To address the variability in sales patterns, the solution employs automated machine learning to perform unsupervised clustering. This technique groups products with similar behaviors and trends for more accurate forecasting. For example, products can be clustered by store location, region, category, or even correlated sales data. This clustering enables the models to make more precise predictions by tailoring forecasting methods to each group’s specific characteristics. Implementing this solution involves integrating AI-driven forecasting systems with the retailer’s existing data sources, such as sales history, promotional schedules, and external factors like holidays or special events. These models can then be trained and refined continuously to adapt to changing market conditions and consumer behaviors. By improving demand forecast accuracy, retailers can optimize their inventory levels, reduce waste, enhance customer satisfaction, and ultimately, boost profitability. For instance, a noted improvement in forecast accuracy can significantly impact profit margins, as demonstrated by a global fashion retailer's increase in profits by several hundred million dollars annually.