Impute Missing Values in a Dataset with Generative AI
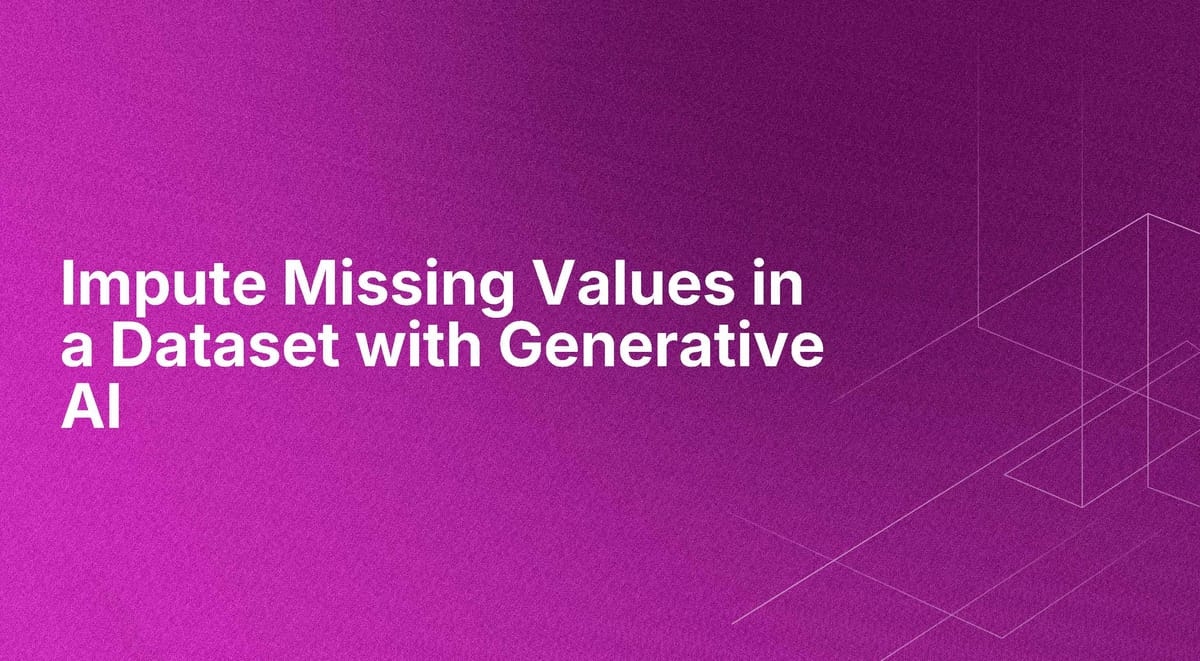
Leveraging AI to Address Data Completeness Challenges
Overview
Data integrity is of paramount importance across industries such as healthcare, finance, retail, and more, where data-driven decisions can significantly impact outcomes. For sectors that rely heavily on predictive analytics and machine learning, the presence of missing data can severely impede the accuracy of models and the validity of resulting insights. AI-driven imputation of missing values is an emerging solution that enhances data quality and model performance, ultimately enabling better decision-making.
Problem Statement
Incomplete data is a common challenge in data analytics and machine learning, leading to biased and less reliable outcomes. When values are missing in datasets, particularly those used for training machine learning models, it creates gaps in the information that the algorithms rely on to identify patterns and make predictions. Traditional methods like averaging or manual imputing can be error-prone and fail to capture the underlying complexities of the data, rendering the subsequent predictions less accurate.
Solution Overview
Generative AI offers a sophisticated approach to imputing missing values by leveraging its ability to detect patterns within large datasets. Unlike traditional methods, AI can utilize complex algorithms to analyze relationships between multiple variables, thereby predicting missing values with higher accuracy. By training on historical data where values are known, machine learning models can learn to estimate missing data points intelligently, maintaining the original data distribution and reducing the risk of introducing biases. On the technical side, implementing generative AI for data imputation requires a pipeline that includes data preprocessing, model selection, and training. Techniques such as k-nearest neighbors, decision trees, or more advanced neural network architectures can be used depending on the nature of the data and the extent of missing values. The model is then evaluated for its performance in predicting missing values, ensuring it generalizes well across various datasets. From a business perspective, this solution not only improves the quality of datasets but also enhances the reliability and accuracy of machine learning models used in critical applications. For example, in healthcare, it can lead to better patient outcomes by improving predictive diagnostic models. In finance, it can lead to more accurate risk assessments and investment strategies. The implementation requires a collaboration between data scientists and domain experts to ensure the imputed data makes logical and practical sense within the specific industry context. Overall, generative AI for data imputation positions businesses to make more informed and confident decisions.