Leveraging Visual AI for Plant Disease Detection
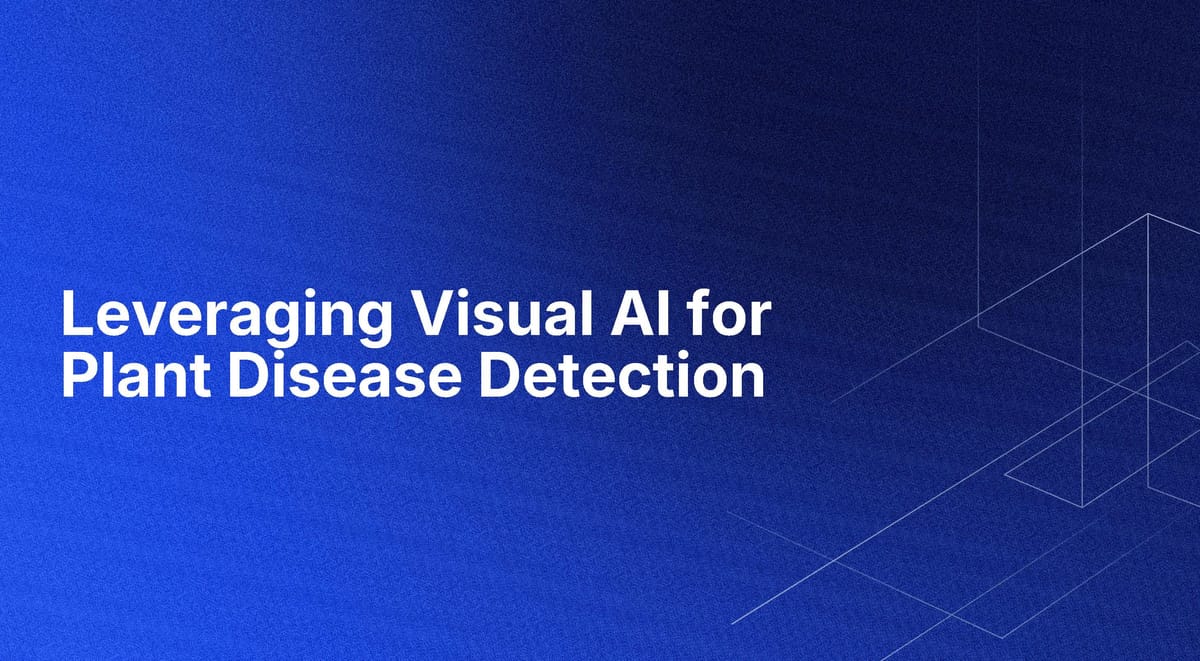
Harnessing Visual AI to Enhance Crop Health and Prevent Yield Loss
Overview
Agriculture is a critical industry that plays a foundational role in supporting the global population. With the anticipated surge to over 9 billion people by 2050, the sector faces immense pressure to increase food production by approximately 70%. One of the major challenges hindering this goal is the prevalence of plant diseases, which can decimate yields and severely impact food security. Leveraging advanced technologies like Visual AI can be transformational in detecting and mitigating plant diseases, providing a sustainable path to achieving higher crop yields.
Problem Statement
Plant diseases are responsible for a substantial decrease in potential crop yields, averaging a 40% reduction, with some farmers experiencing total crop loss. The challenge is particularly acute in developing countries, where access to expert diagnosis and intervention is limited. Timely and accurate detection of plant diseases is crucial to implement necessary measures to curb the spread and minimize impact. However, traditional methods are often costly, time-consuming, and infeasible for smallholders.
Solution Overview
To address these challenges, leveraging Visual AI through mobile applications can provide a game-changing solution. By utilizing the widespread distribution of smartphones, a visual AI model can be developed to diagnose plant diseases from images of plant leaves. The AI model works by learning from thousands of example images, training itself to identify patterns correlated with different diseases or healthy plants. This model is then capable of making accurate predictions when presented with new images, enabling farmers to quickly and effectively diagnose issues in their crops. The implementation involves developing an intuitive mobile application where farmers can capture images of their plant leaves. These images are then processed through the Visual AI model to diagnose the disease or determine the plant's health. The system can provide recommendations for treatment, helping farmers take immediate corrective action. This not only augments the knowledge of smallholders but also acts as an educational tool where farmers can understand what aspects of the plant the AI model focuses on to make its diagnosis. Moreover, this AI-driven approach doesn't require an enormous database of labeled images, as the models can continuously evolve with the addition of new data over time. This continuous learning loop ensures that the system remains relevant and accurate as new diseases emerge and environmental conditions change. By democratizing access to advanced diagnostic tools, Visual AI empowers farmers globally to improve their crop management practices, thereby contributing to increased food security and sustainable agricultural practices.