Predict Churn for Clinical Trials
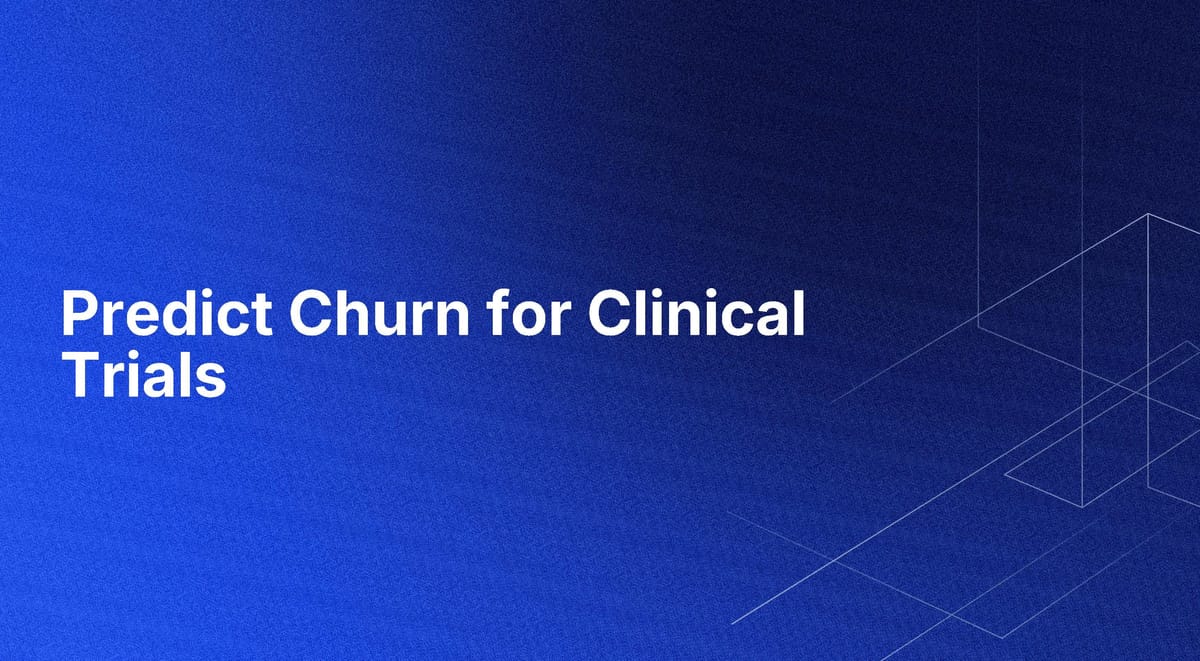
Leveraging AI to Enhance Patient Retention and Reduce Costs in Clinical Trials
Overview
The pharmaceutical and biotechnology industries are cornerstone sectors devoted to drug discovery and development. These industries invest heavily in extensive research and development processes to ensure that new drugs are both safe and effective. A critical phase in this pipeline is clinical trials, which can be exorbitantly expensive and time-consuming. Clinical trials are essential for testing a drug’s efficacy and safety in human subjects, and achieving a successful trial outcome is of paramount importance.
Problem Statement
One of the significant challenges in clinical trials is patient churn, which refers to the dropout of participants before the completion of the trial. This dropout can lead to substantial obstacles, including increased costs, extended timelines, and potentially biased outcomes. Furthermore, the current process for obtaining and retaining patient consent is limited, often emphasizing the ease of withdrawal over sustained participation. These issues culminate in a serious risk to the feasibility and reliability of clinical trial results.
Solution Overview
By integrating AI into the clinical trial process, organizations can accurately predict which patients are most likely to churn and take preemptive measures to mitigate this risk. AI's classification features can analyze vast amounts of data to identify patterns and factors associated with high churn rates. These predictive analytics can then be used to select candidates who exhibit characteristics indicative of lower churn probability. This targeted selection not only helps in retaining patients but also optimizes the pool of trial participants, making the trials more efficient and reliable. Implementation of AI involves training machine learning models on historical clinical trial data to understand the attributes and behaviors that correlate with patient dropout. These models can be integrated into the existing patient management systems to provide real-time risk assessment. Business-wise, this approach can significantly lower the costs associated with patient recruitment and retention while increasing the success rates of clinical trials. Furthermore, reducing patient heterogeneity through advanced classification techniques ensures a more homogeneous group of participants, enhancing the reliability of trial outcomes. Using AI to predict and manage patient churn embodies a transformative business strategy, enabling pharmaceutical companies to execute more successful and cost-effective clinical trials.