Predict Member or Employer Disenrollment
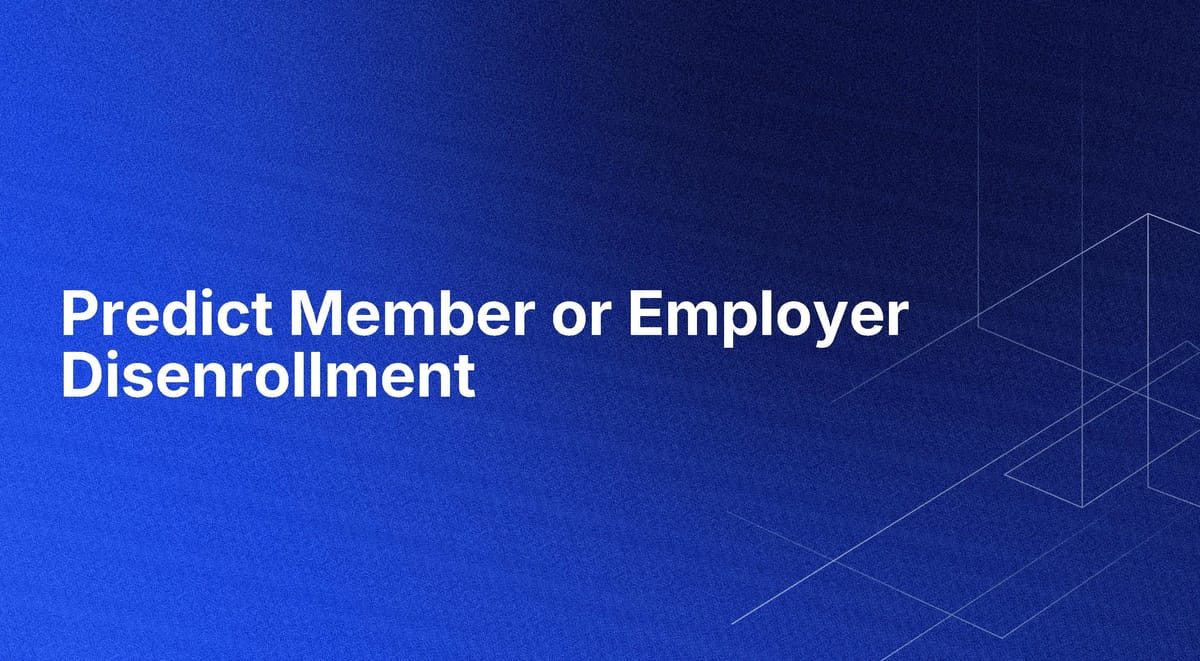
Reducing Member and Employer Churn in Healthcare Through Predictive AI
Overview
The healthcare insurance industry plays a crucial role in the health management of individuals and groups by offering various plans and programs. Insurance payers strive to maintain continuous engagement with their members and employers to ensure consistent premium inflows and effective disease prevention strategies. However, the industry faces significant challenges when members or entire employer groups disenroll from their plans, causing disruptions in care coordination and financial instability.
Problem Statement
Disenrollment from healthcare plans results in severe consequences for both the members and the payers. Members who churn often skip doses or discontinue medications, leading to preventable health issues and increased healthcare costs. For payers, disenrollment translates to reduced premium revenues and a breakdown in managing members' health effectively. Disenrollment, especially at the employer level, exacerbates these challenges, affecting larger groups of members simultaneously.
Solution Overview
Leveraging AI for predicting and reducing disenrollment offers a strategic approach to this industry-wide challenge. AI algorithms can be trained on historical churn data and associated characteristics to identify patterns and signals indicating a higher likelihood of churn for both individual members and employer groups. By utilizing machine learning models, healthcare payers can generate forward-looking predictions on which members or employer groups are at risk of disenrolling. This proactive identification allows for timely intervention strategies tailored to the specific needs and conditions of the at-risk entities.