Predicting Claims Severity from First Notice of Loss (FNOL)
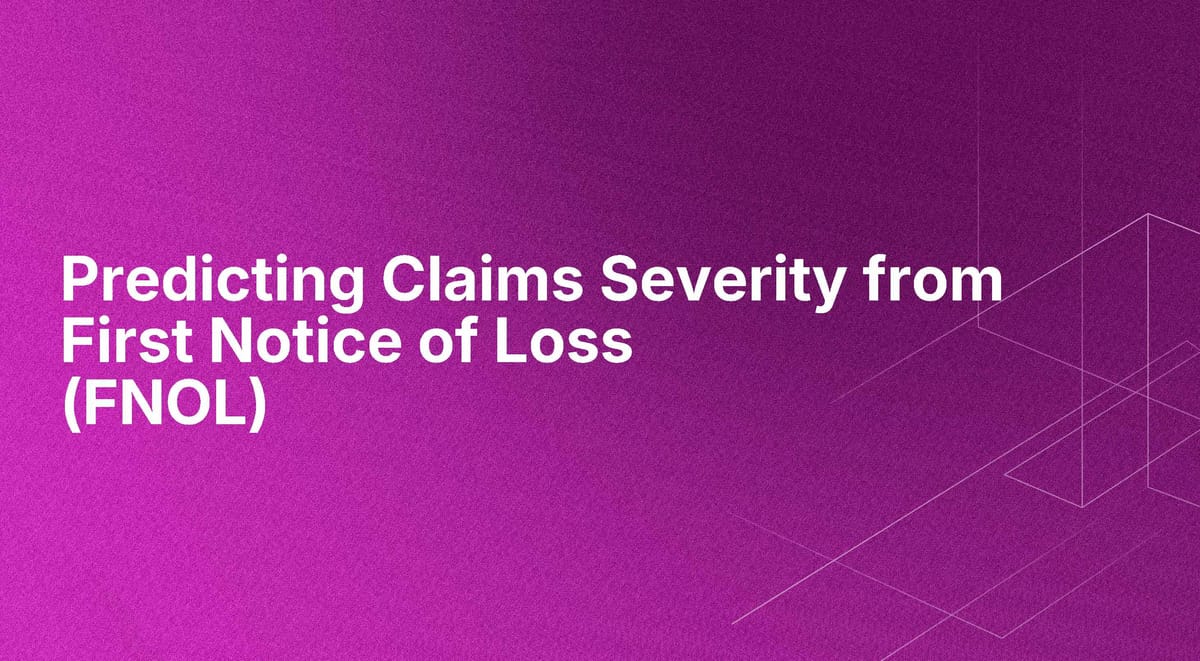
Accurately Estimate Ultimate Payouts for Insurance Claims at FNOL Using AI
Overview
In the insurance industry, particularly in long-tail lines of business such as workers' compensation, claims can take years to be fully paid. Insurers must maintain sufficient reserves for such long-duration claims to ensure they can meet their liabilities when they are due. Accurate prediction of the ultimate payout at the First Notice of Loss (FNOL) is crucial for effective financial planning and operational efficiency within insurance companies.
Problem Statement
Claim payments are typically the largest cost for insurance companies, and unpaid claims constitute a significant liability. The challenge is that the full cost of a claim may not be apparent for many years, making it essential to estimate case reserves accurately at FNOL. Manual processes used by claims adjusters, which rely on personal experience and static guidelines, often lead to less accurate case reserves and inefficiencies, resulting in higher loss adjustment expenses.
Solution Overview
Leveraging AI for predicting claims' ultimate payouts at FNOL can significantly enhance the accuracy of these predictions. By learning complex patterns in historical claims and policy data, AI models can forecast the ultimate claim cost with greater precision than manual methods. This improvement enables claims departments to triage and handle claims more efficiently. For instance, based on the predicted severity, claims can be routed to junior or senior adjusters, or even processed automatically through straight-through processing (STP). This smart allocation reduces the number of claims that escalate to litigation and mitigates overall claim severity. In some cases, up to 60% of claims can go through STP, substantially decreasing operational costs. The implementation of AI in predicting claims severity involves several steps. Initially, a comprehensive dataset containing historical claims and policy details is required to train the model. The AI system uses this data to recognize intricate patterns and correlations that might not be evident through manual analysis. Post-training, the model provides claims adjusters with statistical insights into each predicted payout, facilitating well-informed decision-making. The deployment phase includes integrating the AI system into the claims processing workflow, ensuring seamless operation and enabling insurers to quickly adapt to this innovative approach. This transformation not only improves operational efficiency but also enhances financial stability by ensuring adequate reserves for future claims.