Predictive Modeling for Neurological Outcomes in Spinal Cord Injuries
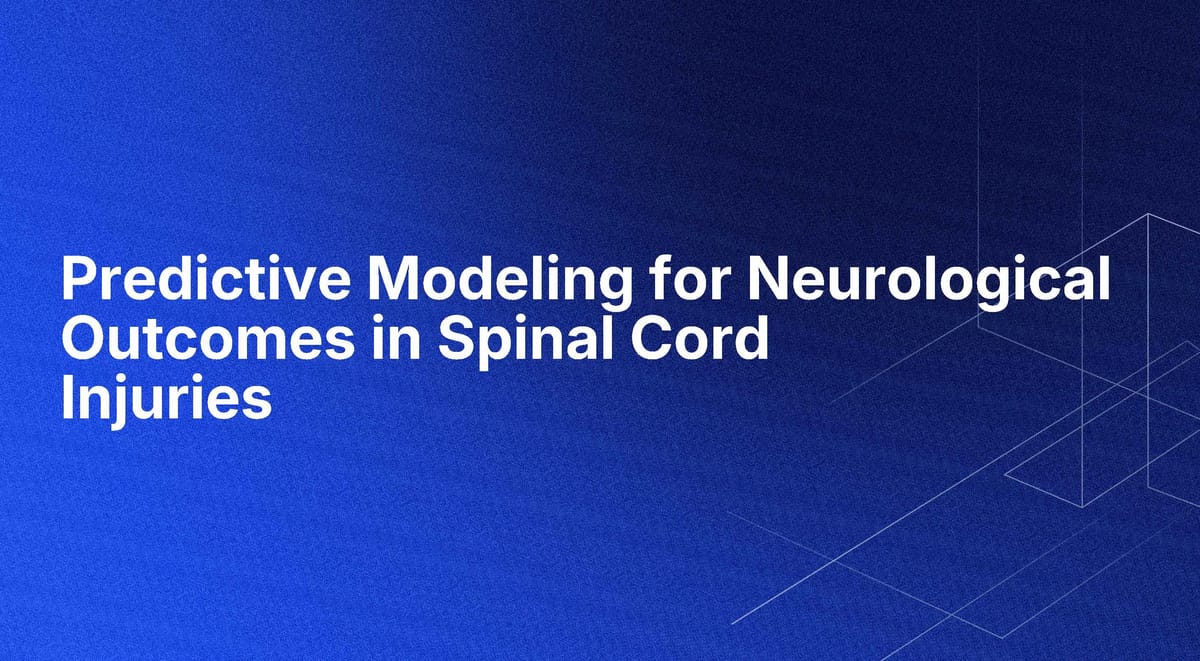
Leveraging Generative AI for Improved Blood Pressure Management in OR
Overview
The healthcare industry is increasingly benefiting from the convergence of advanced technologies to improve patient care and outcomes. In particular, predictive modeling and generative AI frameworks are offering transformative solutions for various medical challenges. One such critical area is the management and treatment of acute spinal cord injuries (SCI), which are rare but profoundly life-altering events. Hospitals and research centers are now utilizing big data and AI to predict patient outcomes, providing invaluable insights for clinical decision-making.
Problem Statement
Acute spinal cord injuries result from sudden traumatic events and have a low rate of complete neurological recovery before hospital discharge. The rarity of these injuries has historically limited opportunities for data-driven research, leading to a lack of comprehensive treatment guidelines. Effective blood pressure management in the operating room (OR) during initial surgery is crucial but the existing guidelines are based on limited empirical data.
Solution Overview
In response to this critical need, UCSF Brain and Spinal Injury Center (UCSF-BASIC), in partnership with Zuckerberg San Francisco General Hospital, has embarked on an ambitious project to leverage AI for predictive modeling. TRACK-SCI, a research initiative under UCSF-BASIC, has amassed the largest dataset to date on acute SCI patients, capturing detailed vital signs data, including systolic and diastolic blood pressure as well as heart rate taken every five minutes during OR procedures. The primary goal is to ascertain if maintaining blood pressure within certain thresholds can predict improvements in neurological function, as measured by the American Spinal Injury Association (ASIA) impairment scale. The predictive model is built using generative AI to analyze patient characteristics, injury details, and derived variables such as the skew and kurtosis of blood pressure readings. Time-series data is scrutinized to explore any clinical relevance of existing blood pressure guidelines and to determine if there are significant upper thresholds. Currently, the models are in the phase of feature reduction, stability analysis, and testing on a prospective dataset for validation purposes. Preliminary findings suggest that time spent in elevated blood-pressure regimes significantly influences the likelihood of neurological improvement. These insights are being meticulously evaluated by clinicians and neurosurgeons to refine treatment protocols, potentially setting new standards for patient care in acute SCI scenarios.