Prioritize Debt Collection by Likelihood of Success
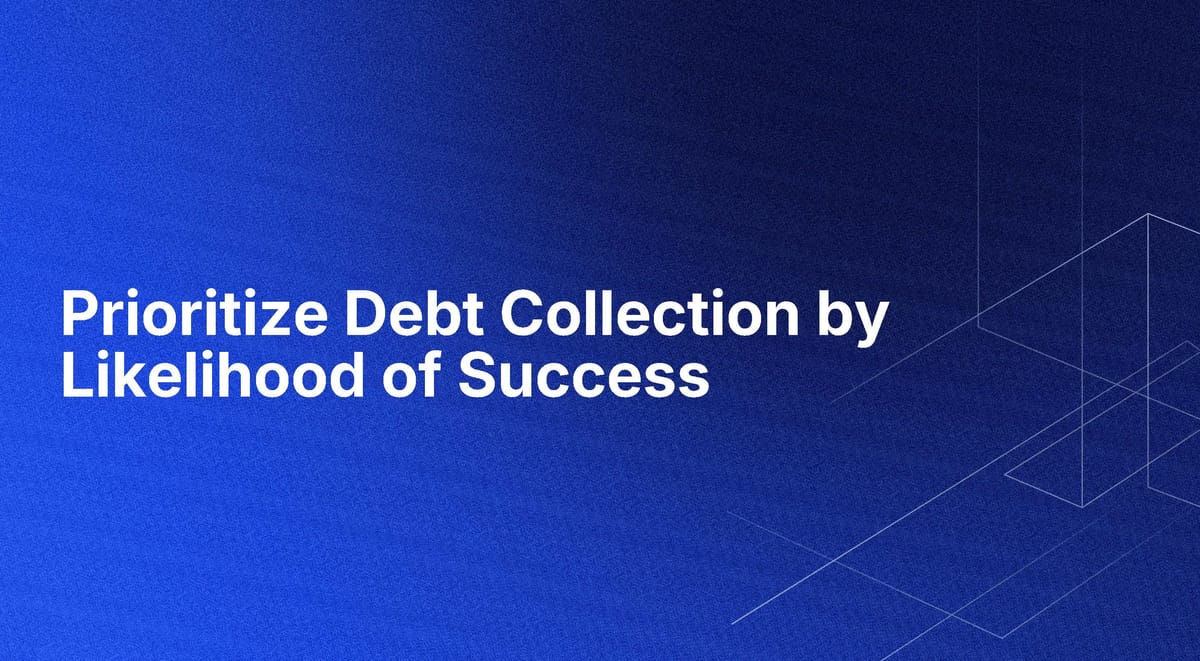
Maximize the effectiveness of debt collection efforts by predicting which borrowers are likely to pay back.
Overview
In the financial industry, interest income remains the primary revenue source. Financial institutions must manage credit risks effectively to maintain a competitive edge, as high default rates can significantly impact their profitability. The ability to accurately assess and manage the risk of borrowers becoming delinquent is crucial. Debt collection is a substantial part of this process, particularly through third-party debt collection agencies that work to mitigate the losses from loan defaults. These agencies currently operate based on historical borrower data without predictive insights for future collections.
Problem Statement
Despite employing meticulous tracking and debt restructuring solutions, third-party debt collection agencies face significant challenges in optimizing their efforts. Around 8% of credit card balances alone fall into default or delinquency within 90 days after their due dates. The current systems lack tools to predict which borrowers are likely to repay, leading to inefficient and sometimes fruitless collections efforts.
Solution Overview
AI-driven solutions offer a transformative approach to address these challenges by leveraging historical data on borrower characteristics. Through machine learning models, AI can predict which borrowers are most likely to repay their debts before collections efforts even begin. This predictive capability allows debt collection agencies to prioritize their resources efficiently, focusing on cases with higher probabilities of repayment and tailoring their strategies accordingly. The AI models analyze a vast array of factors, generating a repayment likelihood score for each borrower. Additionally, they provide insights into the key reasons behind these predictions. This enables debt collectors to customize their communication and approaches based on individual borrower circumstances, fostering more personalized and constructive interactions. For implementation, this solution would involve integrating AI algorithms with existing debt collection systems. Historical data on debt collections would need to be collected, cleaned, and fed into the AI model to train it effectively. Over time, as more data is gathered, the model's predictive accuracy would continue to improve, ensuring that debt collectors have increasingly reliable tools at their disposal. By prioritizing outreach and personalizing strategies, financial institutions could see a notable reduction in delinquencies and an overall improvement in their collections process.