Reducing False Positives in Anti Money Laundering (AML)
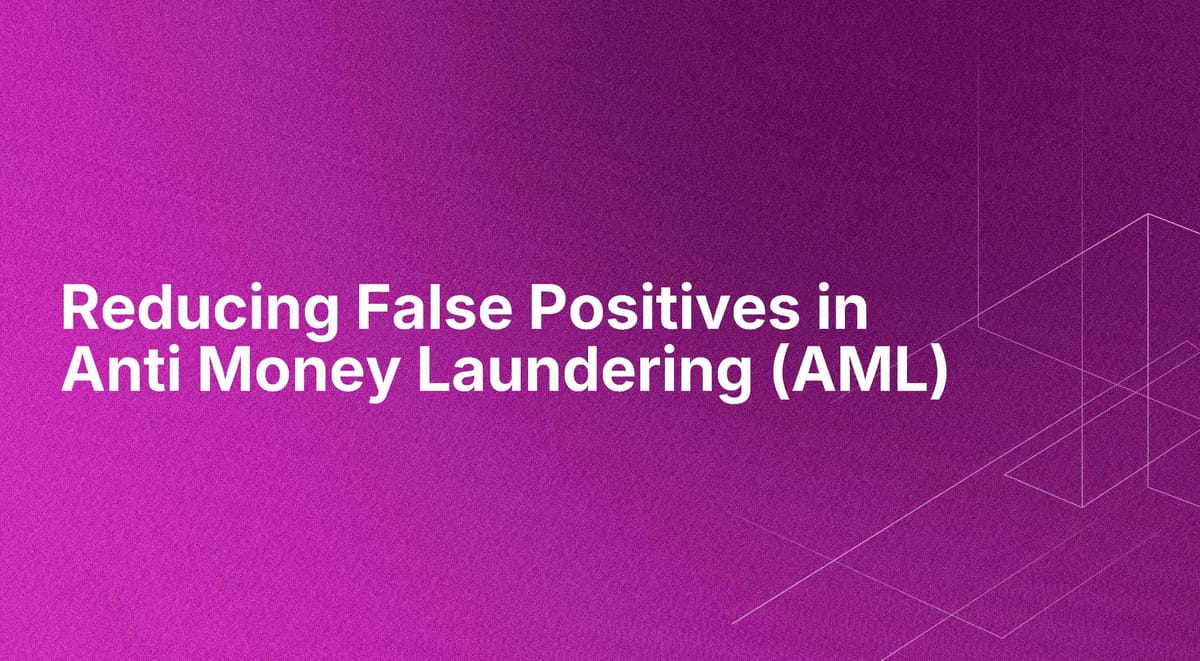
Employing AI to Enhance the Efficiency and Accuracy of AML Transaction Monitoring
Overview
The financial industry is at the forefront of leveraging advanced technologies to combat illegal activities such as money laundering. Regulatory bodies mandate that financial institutions monitor transactions for suspicious activities and file Suspicious Activity Reports (SARs) when necessary. Effective Anti Money Laundering (AML) programs are critical for compliance and the prevention of financial crimes, but traditional methods present significant challenges.
Problem Statement
Current AML systems in the banking sector predominantly utilize rule-based transaction monitoring systems. These systems, while systematic, generate a high volume of false-positive alerts—cases wrongly flagged as suspicious—which can surpass 90%. The excessive false positives result in large workloads for investigation teams and decrease the efficiency of identifying genuine money laundering activities.
Solution Overview
The integration of AI in AML processes offers a transformative approach to mitigate the high false-positive rates and improve overall investigation efficiency. AI models can dynamically learn and recognize complex patterns within transactional data, emulating human intuition in identifying potentially suspicious activities. When deployed, these AI models score and rank new cases based on their risk levels. Cases exceeding a predetermined threshold are escalated for manual review, while those below can be disregarded or subjected to a lighter scrutiny. This prioritization ensures that investigation teams focus their efforts on high-risk cases, thereby utilizing their resources more effectively. AI's ability to continuously retrain and adapt to new data enhances its effectiveness over time. Feedback from investigators about the outcomes of reviews feeds back into the system, enabling it to identify novel money laundering behaviors and improve its accuracy further. This ongoing learning process not only reduces the number of false positives but also ensures that the system evolves with changing patterns of financial crime. Implementing AI within AML frameworks involves initial integration with existing systems and gradually incorporating it into the transaction monitoring and investigation workflows. Training the AI models on historical transaction data to identify patterns that led to SAR filings allows for a robust foundation. As the system operates, continuous refinement based on real-time feedback ensures its efficiency and accuracy are maintained, delivering significant operational cost savings and better compliance with regulatory requirements.