The Future of AI in Healthcare - Treatment, Diagnostics, Ethics, Challenges in Software Development
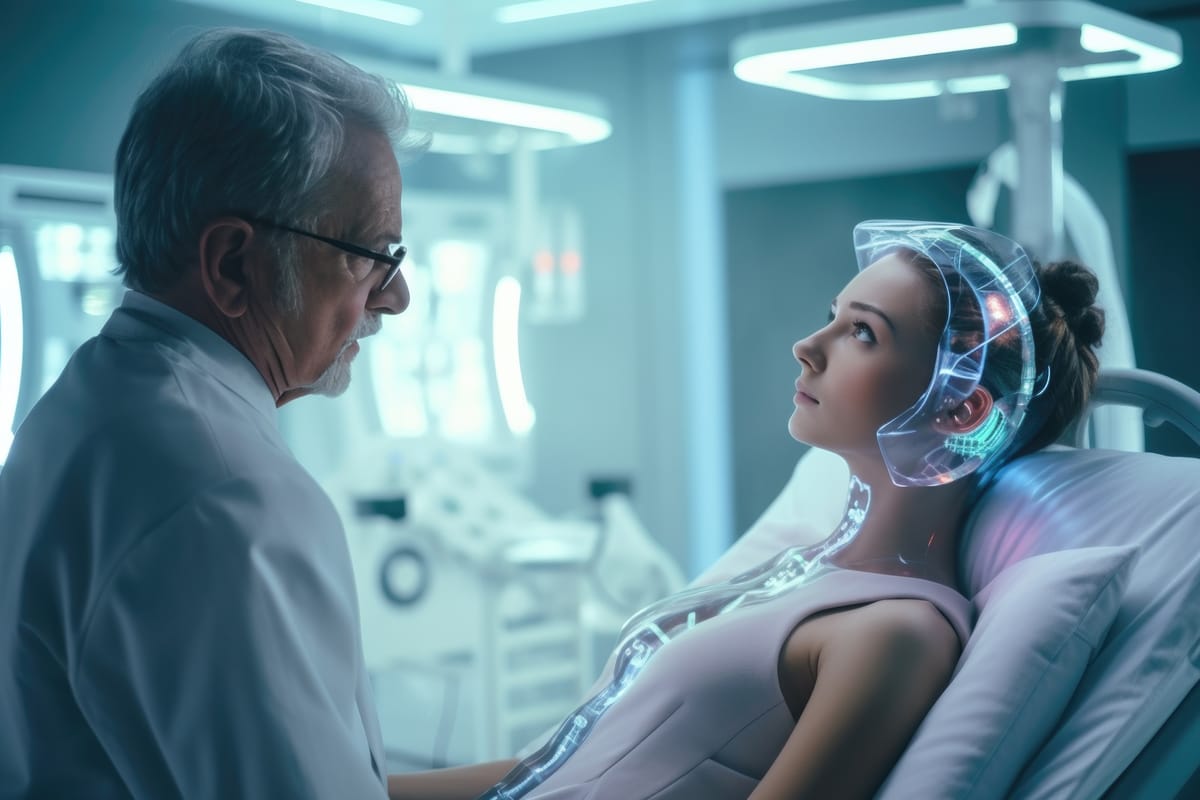
The potential impact of AI
AI will dramatically change healthcare. It will boost efficiency, improve patient outcomes, and enable new treatments and diagnostics.
The future of AI in healthcare includes streamlining administrative tasks, cutting costs, and improving resource use. AI can streamline healthcare admin tasks, cut costs, and improve resource use. For instance, AI systems can analyze vast data faster than any human. This leads to quicker, more accurate diagnoses.
Also, AI can forecast patient admissions. It can detect health crises before they happen. This enables healthcare providers to take a more proactive approach to managing patients. As a result, patient care improves. Emergency room visits and hospital stays drop. This eases the burden on healthcare facilities.
How AI is revolutionizing patient care and diagnostics
AI is not just a data management tool. It is also changing how we approach patient care and diagnostics. Through advanced algorithms and machine learning techniques, AI systems can:
- Suggest personalized medicine. Use the person's genes, lifestyle, and health history.
- AI is not just a data management tool. It is revolutionizing patient care and diagnostics.
- Monitor patient health in real-time using wearable tech. Send alerts if the data suggests a health issue.
These advancements ensure that every patient gets care tailored to them. This could improve treatment efficacy and outcomes.
Branches of medical practice are most likely to replace the first
The future of AI in healthcare will likely impact some areas of medicine more than others. This is especially true for fields with high data-processing needs. It also applies to those with routine, repetitive tasks. These include:
- Radiology: AI can analyze X-rays and MRI scans faster and more accurately than humans.
- Pathology: AI tools can quickly sort cell samples and find flaws.
- Dermatology: AI image recognition can detect skin cancers and other skin conditions without human help.
AI will likely change how specialists work. It aims to boost their efficiency and accuracy, not replace them. As AI evolves, its use in healthcare will spark a new era of innovation and better patient care.
Generative AI in Healthcare
Generative AI relevance to healthcare
Generative AI refers to AI technologies, especially machine learning models. They can create new content based on patterns in vast datasets. In healthcare, this is transformative. It enables complex simulations, predictions, and decisions that mimic human expertise, but faster. This AI can lead to breakthroughs in treatment planning, drug discovery, and personalized medicine.
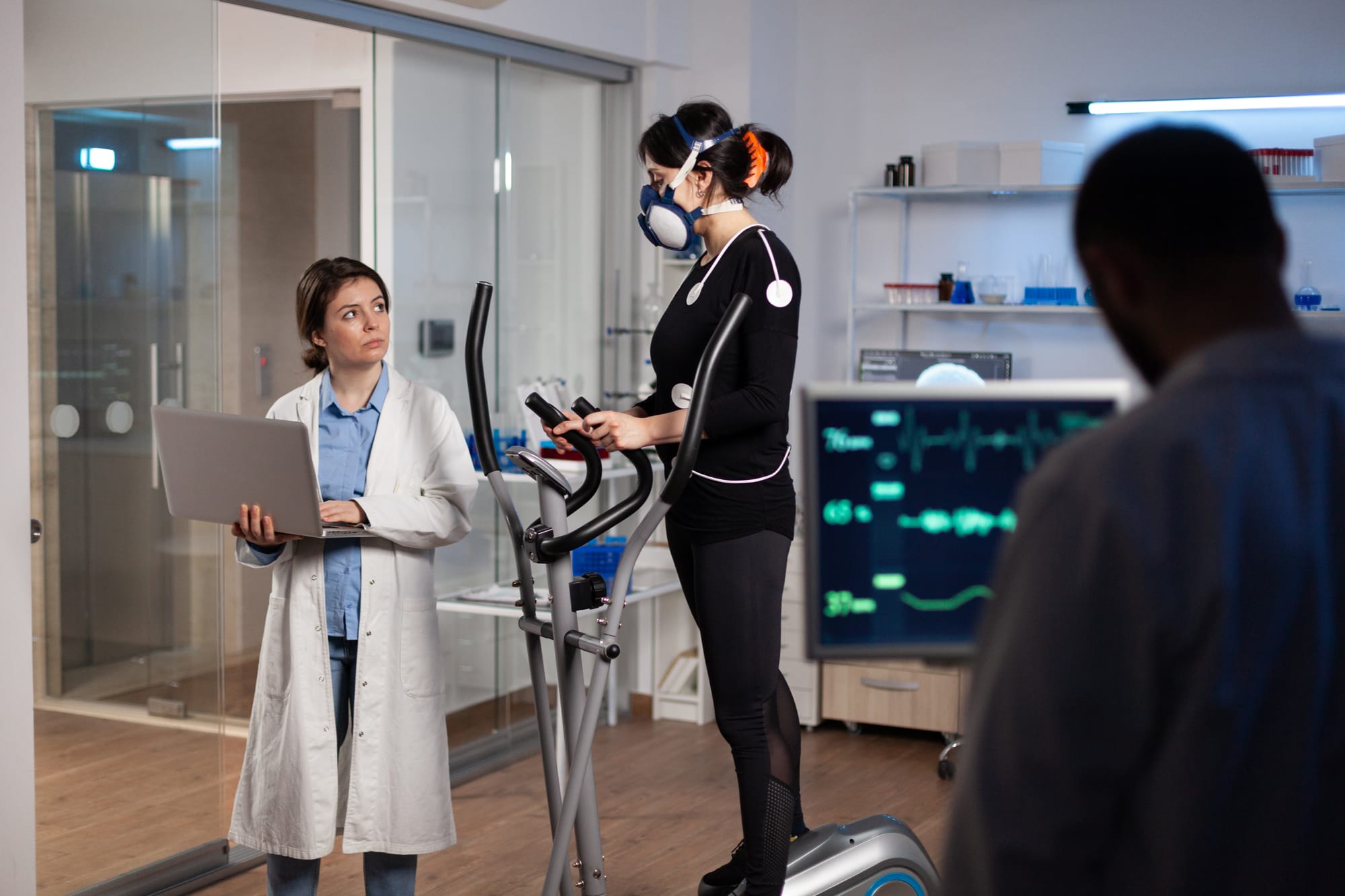
Generative AI in creating synthetic medical data for research
A key benefit of generative AI is its ability to create synthetic medical data. This is vital where real patient data is restricted due to privacy concerns or a lack of data. AI-generated data can help researchers.
It can test hypotheses, validate drug efficacy, and train other AIs. It will not compromise patient confidentiality. Also, this data can be scaled as needed, giving research greater depth and robustness.
Generative AI-tailored treatment strategies
Generative AI is vital to personalized medicine. It helps create tailored treatment strategies. AI can analyze vast patient data, including genetic info and medical histories. It can then design individual treatment plans. These strategies maximize effectiveness and reduce side effects, meeting each patient's specific needs.
How Generative AI improves medical imaging and diagnostics
AI transforms medical imaging, yielding more accurate diagnoses. It can interpret and analyze images with superhuman precision. For example, AI algorithms detect anomalies in X-rays and MRIs. They often spot subtle cues that escape human eyes.
Real-world examples include Google's DeepMind AI. It pinpoints breast cancer in mammograms with precision. IBM Watson helps diagnose and plan cancer treatments. It does this by processing vast medical literature.
Ethical issues of generative AI in healthcare
However, the adoption of generative AI in healthcare also introduces several ethical concerns. Data privacy, consent, and bias in AI models are major issues. We must ensure that AI does not worsen health disparities or discriminate against any groups. AI decision-making processes must be transparent to maintain trust in patient care.
Examples of AI in Healthcare
AI-powered tools for early disease detection
AI diagnostic tools can analyze vast medical data, like images and genetics. They are now faster and more accurate than ever. AI can detect early signs of diseases like cancer and Alzheimer's in scans and tests, often before any symptoms appear. This early detection is crucial for improving treatment outcomes and patient survival rates.
AI used to predict patient outcomes
AI's predictive analytics use past data and machine learning to forecast patient outcomes. This is vital in critical care and surgery. AI models predict complications, hospital readmission rates, and long-term recovery. Such predictions let providers better target interventions and allocate resources.
Use of AI in enhancing surgical precision
Robotic surgery, augmented by AI, is revolutionizing the operating room. These AI systems give surgeons better visuals, dexterity, and precision beyond human limits. As a result, surgeries become less invasive, with reduced recovery times and decreased risk of infection. This not only benefits patient safety but also improves surgical outcomes.
AI's role in accelerating the development of new medications
AI greatly speeds up drug discovery and testing. These are slow, costly tasks. AI can quickly screen thousands of molecules. It can predict their interactions with human cells. This can identify promising drug candidates much faster than traditional methods. It also helps design clinical trials and predict side effects. This makes drug development more efficient and safer.
AI-based systems to streamline healthcare operations
AI-based systems are key to streamlining healthcare operations. They handle tasks like scheduling, medical records, and insurance claims. These systems reduce admin work, cut costs, and improve services. They let healthcare pros focus on patient care instead of paperwork. Such efficiencies are vital for scaling healthcare to meet rising global demand.
Challenges in Software Development for AI in Healthcare
Generative AI with Older Healthcare Technologies
Integrating AI into existing healthcare systems with outdated tech poses major challenges. Older systems often can't handle the high-speed, high-volume data processing that advanced AI needs. This can cause compatibility and efficiency issues.
Obtaining High-Quality Medical Data for training AI models
High-quality, diverse medical data is crucial for training effective AI models. However, it often involves complex privacy issues and consent requirements. It may also be fragmented across different healthcare providers. This complicity makes data acquisition hard and reduces the accuracy of AI applications.
Complexities in Ensuring AI Systems Comply with Healthcare Laws
AI systems must follow strict healthcare laws. Regulatory frameworks like HIPAA safeguard medical data in the U.S. They protect its privacy. Balancing AI innovation with these evolving laws poses challenges. Developers must align AI solutions to current privacy standards. This delicate dance between progress and protection demands constant scrutiny. As AI capabilities surge, careful navigation of the regulations is crucial. There are also challenges in scaling AI to work in various healthcare settings.
Scaling AI solutions across different healthcare settings is often problematic. Each has a unique workflows. AI systems must be adaptable and robust. They must meet the diverse needs of various settings, from large hospitals to small clinics. This often requires extensive customization and rigorous testing.
Need for Collaboration Between Software Developers, Healthcare Professionals, and Ethicists
Effective AI deployment in healthcare needs a team effort. It must involve software developers, healthcare professionals, and ethicists. This collaboration ensures that tech advancements meet clinical needs and ethical standards. It will lead to innovations that are both effective and socially responsible.
AI vs. Medical Doctors
As the future of AI in healthcare advances, it's clear that AI is a tool to enhance, not replace, medical professionals. That's a far-fetched idea. AI is best viewed as a tool to enhance medical professionals, not replace them. Doctors provide empathetic care. They understand human nuances. They make complex judgments that AI cannot replicate. Instead, AI will support and improve decision-making. It will give doctors deeper insights.
Conclusion: The Future of AI in Healthcare - A Balanced Perspective
AI in healthcare offers faster diagnoses, personalized treatments, and disease prevention. However, we must balance innovation with ethics and patient care. We can use AI responsibly by addressing data privacy, biases, and inclusivity. This will enhance and secure the future of AI in healthcare, improving human skills without replacing them.